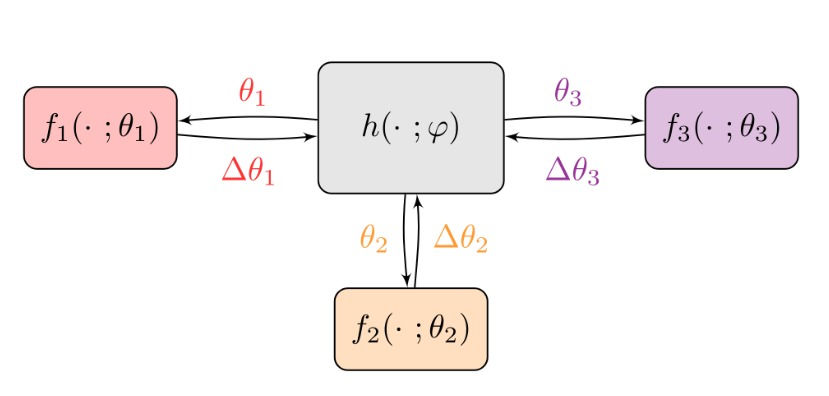
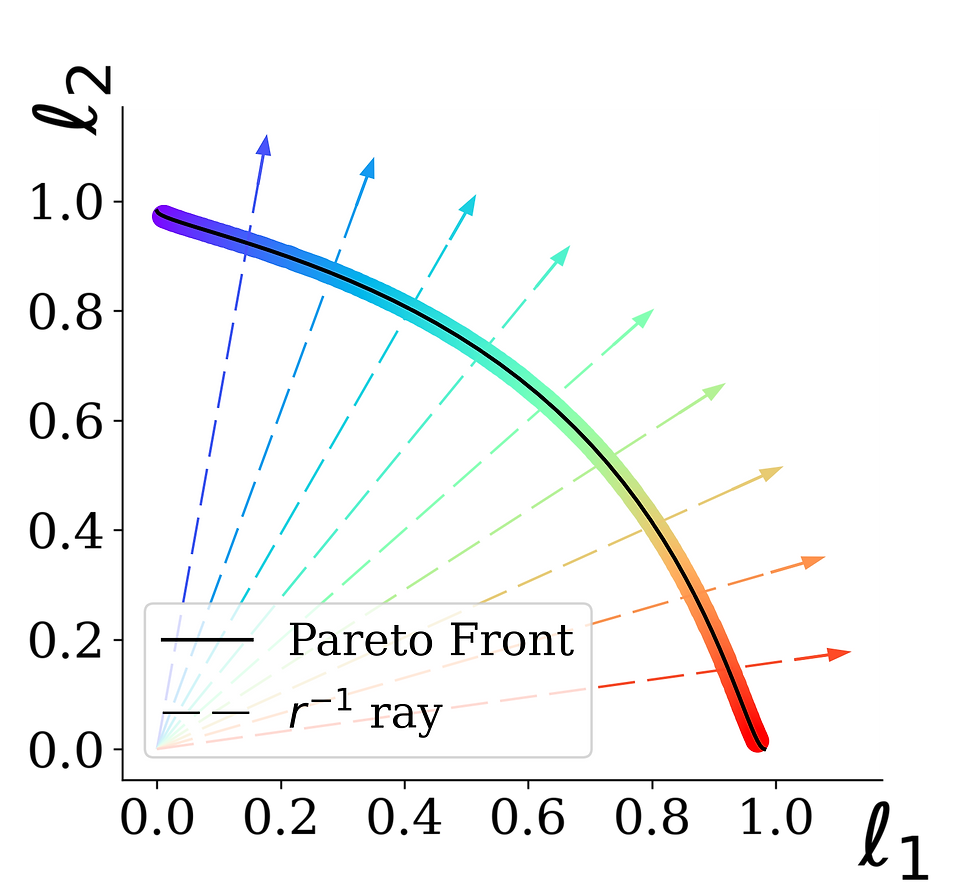
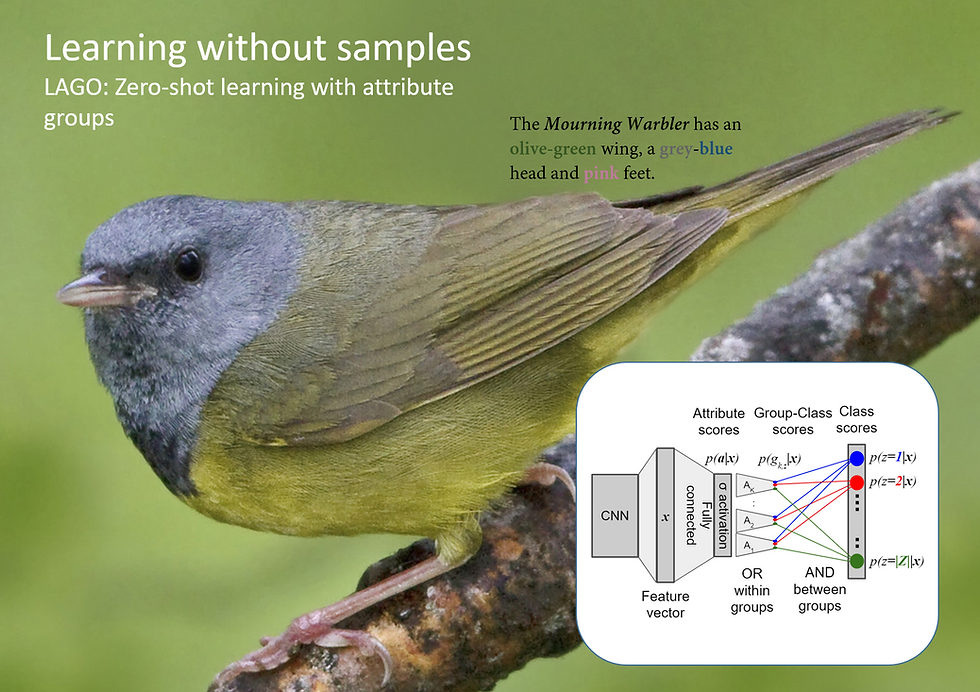
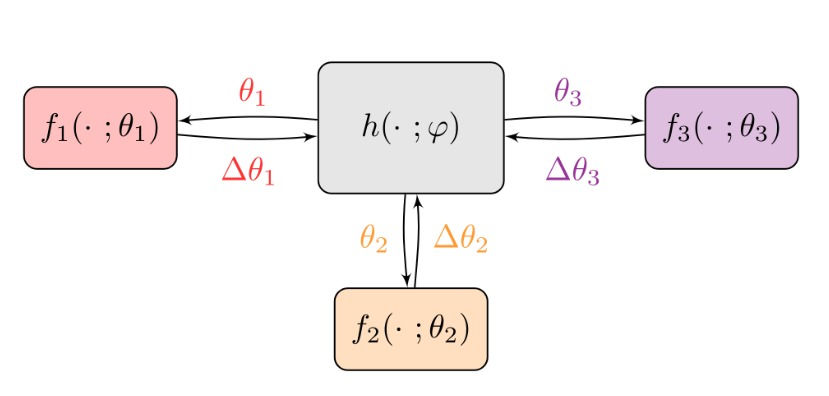
We study learning in brains and machines.
To teach machines to generalize from examples, we develop algorithms to represent complex signals in meaningful ways. We teach our machines to understand sounds and images, generate them, and reason about them especially in complex scenes and with few samples.
We draw inspiration from biological systems. Brains develop and change following experience. People learn even with only few examples, and can reason and generalize in diverse novel scenarios.
Learn with few or no samples, compositional
ICCV2021 UAI2018, CVPR2019 WACV2021 NeurIPS2020,
UAI2021 ECCV2022, ICLR2023 ...
Generative Visual AI: ICLR 2023, NeurIPS 2023, AAAI2024
Learn multiple tasks : ICLR 2021 NeurIPS 2021, ICML2023 ICML2022, ICML2023
Reasoning about visual scenes ECCV2022 CVPR 2017, CVPR 2017, Compo', CVPR2019, ICCV2019, WACV2020, ECCV2020
Learning in structured domains graphs, sets, deep-models
NIPS 2018, WACV2020, ICML 2020 (best paper), ICML2021 ICML2021, ICML2023, ICML 2024, ICML2024
Brain:
How does gene expression change during brain development?
PLoS-CB 2013, PLoS-CB 2015, PLoS-CB 2013
How do neurons represent sounds? Neuron 2006, PNAS 2012